How to Deploy AI Agents Safely and Responsibly in Banking
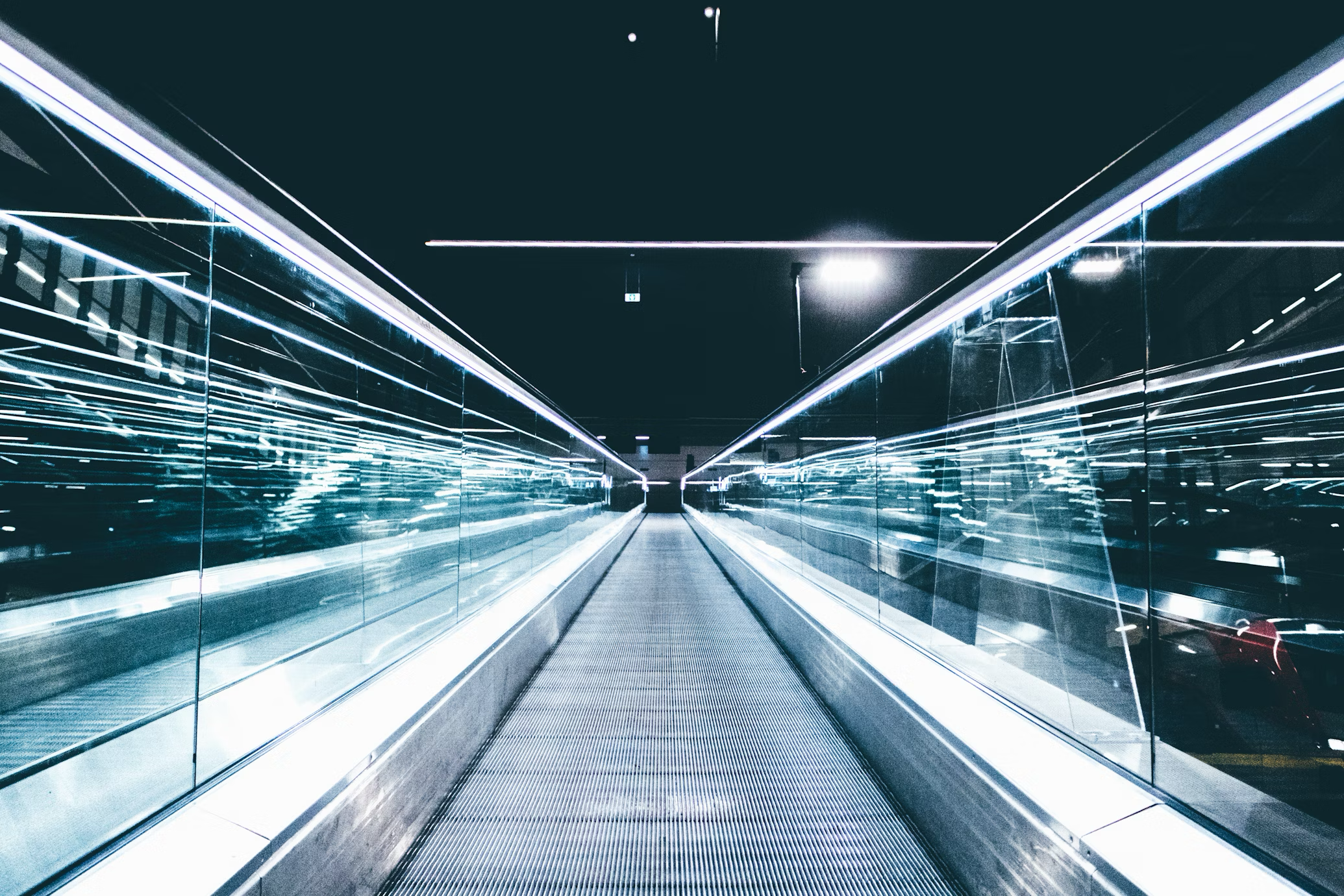
How to Deploy AI Agents Safely and Responsibly in Banking
The Opportunity and the Obligation
AI agents are no longer a futuristic concept — they are being deployed today to automate tasks, support decision-making, and personalize services across the banking sector. But with great potential comes great responsibility.
In regulated industries like banking, deploying agentic AI isn't just about getting the technology right — it's about ensuring that these systems are safe, explainable, compliant, and aligned with institutional values and customer trust.
This blog explores the key steps, considerations, and best practices to deploy AI agents in a safe, responsible, and impactful way.
1. Define the Role and Boundaries of the Agent
Every AI agent needs a clear job description. What task is it performing? What decisions is it allowed to make? Where does human oversight kick in?
Start by defining:
- The use case and business objective (e.g., onboarding, fraud detection, customer support)
- The level of autonomy (suggest vs. decide vs. act)
- The rules and constraints under which the agent must operate
For example, a customer onboarding agent might be allowed to pre-fill forms, validate uploaded documents, and provide real-time support — but not approve accounts, which would be escalated to a human.
Clearly defined roles prevent scope creep, ensure compliance, and reduce organizational resistance. Teams know what the agent will and won’t do, helping align expectations and accelerate deployment.
2. Ensure Data Privacy and Governance
AI agents rely on large volumes of sensitive data to function effectively — but this data must be used responsibly and ethically.
To ensure privacy:
- Follow data minimization principles: only access what’s needed
- Apply anonymization or tokenization when possible
- Track and log data lineage and provenance
- Enforce role-based access control for all data operations
For example, a financial health coaching agent could generate insights from transaction data, but should not store raw account details or share them outside the session.
Strong data governance builds customer trust, ensures regulatory compliance, and minimizes the risk of breaches or fines — which are particularly costly in finance.
3. Build for Explainability and Auditability
Banking systems must be explainable to regulators, auditors, and end users. If an agent takes an action or makes a recommendation, it should be clear why.
Best practices:
- Use transparent reasoning frameworks (e.g., decision trees, chain-of-thought prompts)
- Record agent decisions, inputs, and tools used for each task
- Enable human-in-the-loop checkpoints in sensitive workflows
For instance, if a compliance agent flags a transaction as suspicious, it should be able to explain the exact pattern or trigger that caused the alert.
Explainability enables trust, speeds up audits, and avoids “black box” risks. It empowers teams to learn from the system and continually improve performance.
4. Implement Guardrails and Monitoring
Autonomy without oversight is risky. AI agents should operate with real-time monitoring and built-in safety nets.
Implement:
- Hard guardrails (e.g., agents can’t approve transactions over $10K)
- Soft guardrails (e.g., escalate if confidence score is low or customer sentiment is negative)
- Ongoing performance monitoring to detect drift, bias, or failure patterns
- Kill switches to deactivate or retrain misbehaving agents
For example, a virtual agent that handles loan prequalification may require escalation if debt-to-income ratios fall outside a tolerable range.
Guardrails mitigate reputational and financial risk. Monitoring ensures the agent continues performing accurately and fairly — even as the environment changes.
5. Test and Validate Extensively
Before deployment, simulate real-world conditions to reduce surprises and prepare for edge cases.
To validate:
- Run stress tests and edge-case scenarios across different personas and inputs
- Use shadow deployments alongside human teams to compare outcomes
- Measure accuracy, consistency, fairness, and latency under load
- Gather feedback from frontline users and compliance teams
For example, test how an agent responds to customers entering incomplete or ambiguous information during digital onboarding.
Thorough testing increases system resilience, builds internal confidence, and reduces post-launch remediation costs.
6. Train and Enable Your People
Agents don’t replace people — they augment them. But for this to work, your staff needs to understand how agents work, when to trust them, and how to intervene.
Key steps:
- Train teams on agent capabilities and limitations
- Establish clear handoff protocols between agents and humans
- Create feedback channels to continuously improve agent behavior
For example, in a contact center, agents could escalate to humans after two failed attempts, and human reps should know how to resume the conversation with full context.
Empowered staff amplify the impact of AI agents. Human-AI collaboration leads to better experiences and reduces fear or resistance among teams.
7. Start Small, Learn Fast, Scale Wisely
Begin with low-risk, high-value use cases where oversight is easy. Prove value, learn from real usage, and then expand to more complex or sensitive domains.
Use an MVP-first approach:
- Start with a narrow agent scope (e.g., FAQ bot or fraud alert assistant)
- Launch in a controlled environment or specific customer segment
- Use data and feedback to refine and iterate
For example, a bank might start by deploying a sentiment-detection agent in one support channel before scaling it across all customer touchpoints.
Starting small reduces risk while building a strong foundation for broader adoption. Fast feedback cycles accelerate innovation and help avoid costly missteps.
Conclusion: Responsible AI Is Strategic AI
Responsible deployment isn’t just about compliance — it’s a competitive advantage. The institutions that deploy AI agents with care, transparency, and governance will gain faster trust, better adoption, and more enduring success.
At symplistic.ai, we help banks and fintechs build and deploy safe, industry-aligned AI agents — from pilot to production. Our approach combines technical rigor with business alignment, ensuring agents work for people, not around them.
Safe, smart, and scalable — that’s the future of AI in banking.
See all our blogs here.